Urban Crisis Simulation
Realtime Urbanism Lab
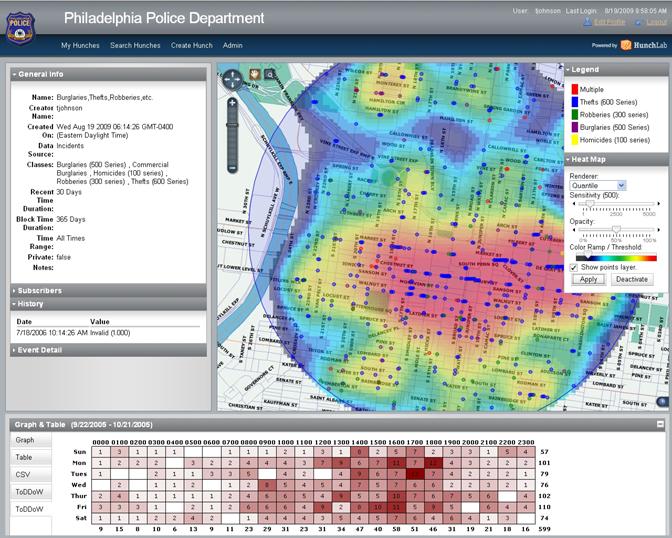
This research, platform, and exhibition project investigates new and recent urban simulation technologies that virtualize urban environments and urban subjects. The project looks at the relationship between the production of urban crises and the implementation of surveillance and simulation technologies. From the New York Police Department’s proprietary predictive policing algorithm to Uber’s demand forecasting and surge pricing algorithm, many spheres of urban life are governed by digital anticipation. What, this project asks, are the records, tools, and methods used to simulate a city for reasons of control and monetization? What are the capabilities of specific technical instruments and infrastructure? And what are the consequences for the built environment and the people who move through it?
The computer models that drive these simulations are based in abstract theories of individual and collective human decision making. These theories are informed by genealogies of knowledge explicitly rooted in social ordering belief systems. Recent scholarship has revealed the eugenic roots of the psychometric analysis and trait psychology that informed Cambridge Analytica’s data-driven personality predictions. Examples such as virtual Iraqis performing non-verbal cues for military sensitivity training in software developed for the US Army, or synthetic populations based on household units traveling through a city’s transportation network for study by traffic engineers at New York City’s Metropolitan Transportation Authority also reveal the bias and assumptions behind these theories. Decision making based on these problematic models produces unfair outcomes that favor those in power. Simulations, and their increasing ubiquity, also raise ethical questions. How can we think about the rights of actual humans when their decisions and movements are rendered as virtual analogs? How do we better advocate for the publics whose gestures, behaviors, and movement patterns have been recorded, quantified, and put into digital motion to drive the very insights that are reinscribed back onto their bodies? These questions are particularly urgent because of virtual human demographics—low-income communities of color, and religious and ethnic minorities are more often the target of urban surveillance and data collection than white Americans.
Methods and Outputs
This project is conducting an extensive survey of urban simulations to examine the implications of their data-collecting practices, mapping systems, and artificial human decision-making models. The research is also examining the blind spots of supposedly all-immersive, all-seeing simulations to understand how such simulations foreclose on urban futures outside the purview of military, security, and financial motives. Importantly, part of the research will look for new ways to resist and undermine invasive and pervasive technologies. For example, much of the information driving predictive models has been collected through opaque channels, what would a data-consenting ecosystem look like and what sort of political channels would need to be pursued to implement new practices. What would it look like if communities were formulating the questions these models ask? We can use urban simulation as a counter cartography, and in a collaborative way with many stakeholders to rehearse the city we want and create a roadmap for how we get there.
This project will result in a global taxonomy of new urban simulation technologies, implementations and practices. This taxonomy will be a rich dataset consisting of “simulations of simulations” made using the very tools and techniques that are being studied.
Project Team
Farzin Lotfi-Jam (Project Director)
Thuan La (Research Assistant)
Desai Wang (Research Assistant)